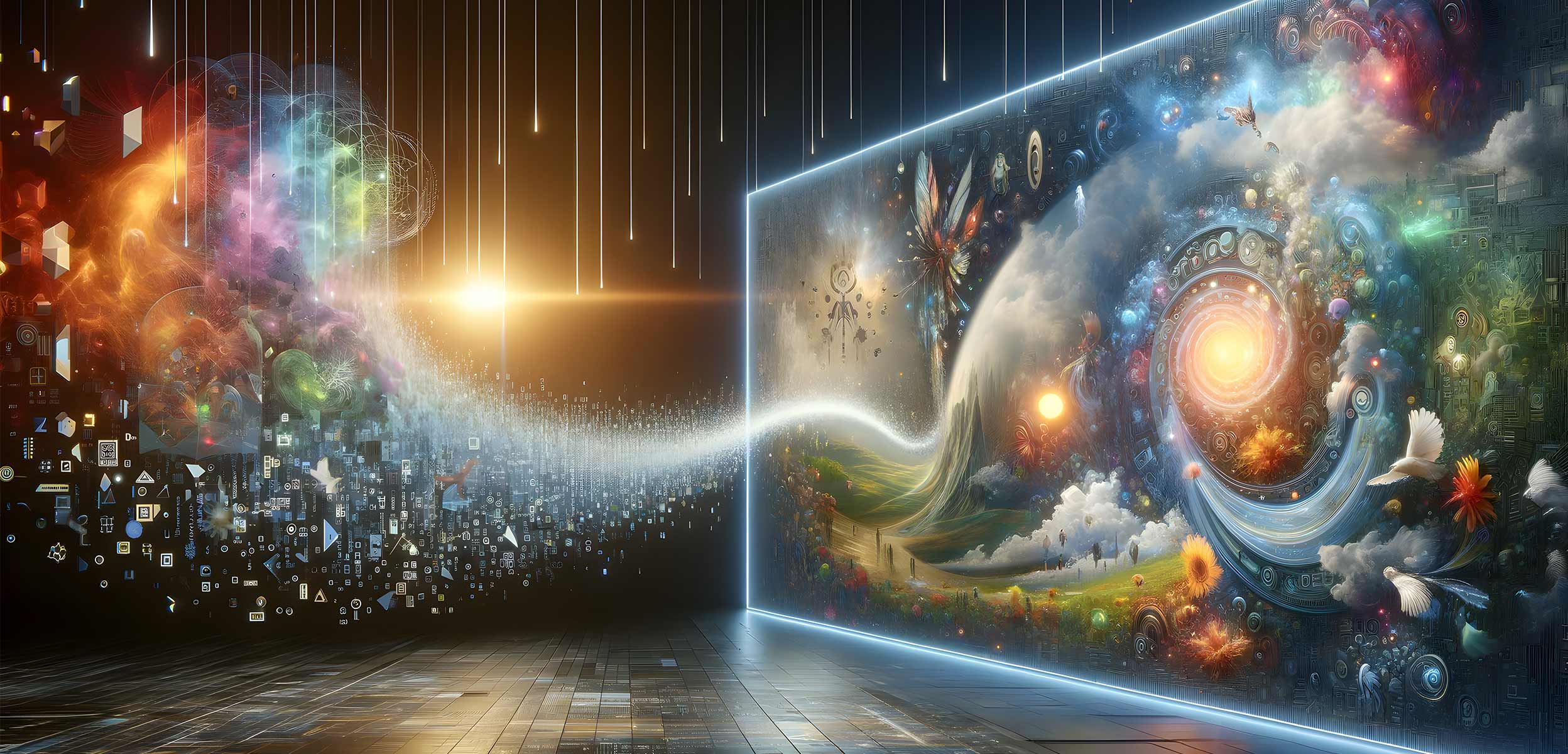
Tools like DALL-E have transformed AI beyond ChatBots, revolutionizing image creation from text. Each of these ImageBots possesses unique strengths and weaknesses. The one excels in generating intricate artistic pieces, the other specializes in crafting highly realistic images. Presented here is a concise overview highlighting some of the most well-known ImageBots.
- DALL-E (OpenAI): Known for its ability to create varied, lifelike images from text descriptions. Though still in development, it’s very promising. Yet, accessibility remains limited. Access can be obtained for instance by signing up for the waitlist to upgrade to GPTplus or through Microsoft Bing Chat and Bing Image Generator. Go to Bing, choose ‘Chat’ in the horizontal menu, then enter the description of the image you want to create.
- Stable Diffusion (Computer Vision team at LMU Munich in collaboration with Runway AI): Focuses on achieving photorealistic image generation from text descriptions. You can explore Stable Diffusion XL by clicking on the following link.
- GauGAN2 (NVIDIA): Generates both lifelike and artistic images from text-based prompts, such as ‘A black cat in an astronaut suit on the lunar surface’. It seems that the GauGAN2 web demo has been taken down from the NVIDIA AI Playground website. Nonetheless, you can watch the video ‘Introduction of GauGAN2 by NVIDIA Research’.
- Leonardo.ai (Leonardo.ai) is an AI image generation tool available for free with certain limitations. Upgrading to a paid subscription offers additional features such as higher-resolution images, enhanced creative control, and access to various styles. It’s a suitable option for individuals interested in creating realistic images without requiring advanced technical skills. Click on this link and try some of the features.
Many AI image generation tools leverage a blend of models, including GANs, WAEs, WAE-GANs, StyleGANs, and Diffusion Models. For those keen on delving deeper into this realm, the following text offers an exploration of the mechanisms of these models.
- GAN, short for Generative Adversarial Network, utilizes two neural networks – the generator and the discriminator. The generator produces new data instances, while the discriminator works on distinguishing between generated and real data. Throughout training, the generator aims to create more realistic data to deceive the discriminator, while the discriminator strives to better discern between real and generated data. This continuous exchange leads to enhancements in both networks until the generated data becomes very realistic.
- WAEs, short for Wasserstein Autoencoders, use an autoencoder-like structure. An autoencoder consists of an encoder and a decoder. The encoder compresses data into a ‘latent representation’, which captures the essence of the input data in terms of its probability distribution. In a WAE, the decoder aims to reconstruct the original data from this compressed representation while ensuring that the rebuilt data follows a probability distribution that closely matches that of the original data. This closeness is often measured using an appropriate distance, frequently the Wasserstein distance, which quantifies the similarity between the two probability distributions.
- By combining ideas of WAEs and of GANs, WAE-GANs aim to benefit from the stability and diversity of sample generation that GANs offer, while also focusing on learning a meaningful latent space representation of the data, as emphasized by WAEs. This combination attempts to address some of the limitations or challenges faced by both GANs and WAEs individually, creating a more robust and effective generative modeling technique.
- StyleGAN’s design enables the independent encoding of style features and content details. This distinguishes StyleGANs from conventional GANs. The capacity to separate style and content attributes is the key aspect behind StyleGAN’s prowess in producing a wide array of realistic images.
- Diffusion Models start with a blurry, noisy image, progressively refining it to become sharper, more intricate, and lifelike. They achieve this by employing a sequence of filters that eliminate noise while retaining the image’s crucial features. This process resembles gradually cleansing a soiled window to unveil an pristine view.